Successfully reported this slideshow.
Data processing platforms architectures with Spark, Mesos, Akka, Cassandra and Kafka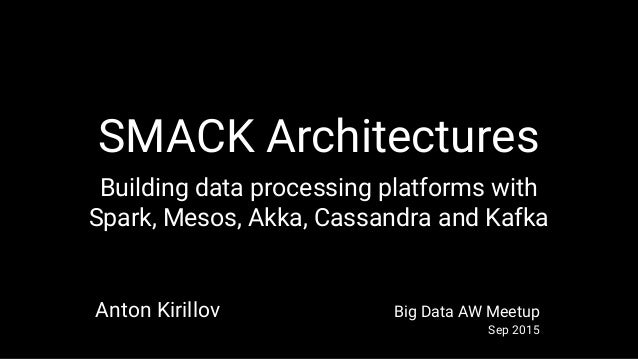

















![Akka features
class JsonParserActor extends Actor {
def receive = {
case s: String => Try(Json.parse(s).as[Event]) match {...](https://www.slideshare.net/akirillov/data-processing-platforms-architectures-with-spark-mesos-akka-cassandra-and-kafka)











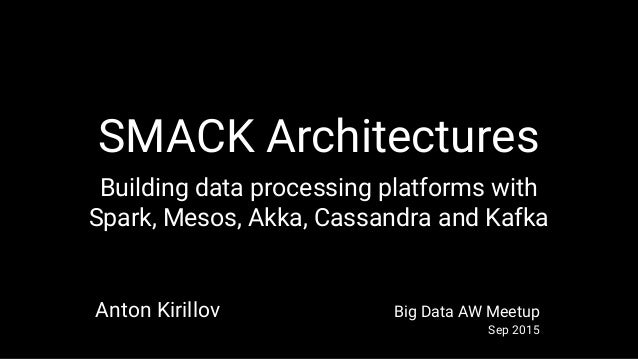
Upcoming SlideShare
Loading in …5
×
- 1. SMACK Architectures Building data processing platforms with Spark, Mesos, Akka, Cassandra and Kafka Anton Kirillov Big Data AW Meetup Sep 2015
- 2. Who is this guy? ● Scala programmer ● Focused on distributed systems ● Building data platforms with SMACK/Hadoop ● Ph.D. in Computer Science ● Big Data engineer/consultant at Big Data AB ● Currently at Ooyala Stockholm (Videoplaza AB) ● Working with startups 2
- 3. Roadmap ● SMACK stack overview ● Storage layer layout ● Fixing NoSQL limitations ● Cluster resource management ● Reliable scheduling and execution ● Data ingestion options ● Preparing for failures 3
- 4. SMACK Stack ● Spark - fast and general engine for distributed, large-scale data processing ● Mesos - cluster resource management system that provides efficient resource isolation and sharing across distributed applications ● Akka - a toolkit and runtime for building highly concurrent, distributed, and resilient message-driven applications on the JVM ● Cassandra - distributed, highly available database designed to handle large amounts of data across multiple datacenters ● Kafka - a high-throughput, low-latency distributed messaging system designed for handling real-time data feeds 4
- 5. Storage Layer: Cassandra ● optimized for heavy write loads ● configurable CA (CAP) ● linearly scalable ● XDCR support ● easy cluster resizing and inter-DC data migration 5
- 6. Cassandra Data Model ● nested sorted map ● should be optimized for read queries ● data is distributed across nodes by partition key CREATE TABLE campaign( id uuid, year int, month int, day int, views bigint, clicks bigint, PRIMARY KEY (id, year, month, day) ); INSERT INTO campaign(id, year, month, day, views, clicks) VALUES(40b08953-a…,2015, 9, 10, 1000, 42); SELECT views, clicks FROM campaign WHERE id=40b08953-a… and year=2015 and month>8; 6
- 7. Spark/Cassandra Example ● calculate total views per campaign for given month for all campaigns CREATE TABLE event( id uuid, ad_id uuid, campaign uuid, ts bigint, type text, PRIMARY KEY(id) ); val sc = new SparkContext(conf) case class Event(id: UUID, ad_id: UUID, campaign: UUID, ts: Long, `type`: String) sc.cassandraTable[Event]("keyspace", "event") .filter(e => e.`type` == "view" && checkMonth(e.ts)) .map(e => (e.campaign, 1)) .reduceByKey(_ + _) .collect() 7
- 8. Naive Lambda example with Spark SQL case class CampaignReport(id: String, views: Long, clicks: Long) sql("""SELECT campaign.id as id, campaign.views as views, campaign.clicks as clicks, event.type as type FROM campaign JOIN event ON campaign.id = event.campaign """).rdd .groupBy(row => row.getAs[String]("id")) .map{ case (id, rows) => val views = rows.head.getAs[Long]("views") val clicks = rows.head.getAs[Long]("clicks") val res = rows.groupBy(row => row.getAs[String]("type")).mapValues(_.size) CampaignReport(id, views = views + res("view"), clicks = clicks + res("click")) }.saveToCassandra(“keyspace”, “campaign_report”) 8
- 9. Let’s take a step back: Spark Basics ● RDD operations(transformations and actions) form DAG ● DAG is split into stages of tasks which are then submitted to cluster manager ● stages combine tasks which don’t require shuffling/repartitioning ● tasks run on workers and results then return to client 9
- 10. Architecture of Spark/Cassandra Clusters Separate Write & Analytics: ● clusters can be scaled independently ● data is replicated by Cassandra asynchronously ● Analytics has different Read/Write load patterns ● Analytics contains additional data and processing results ● Spark resource impact limited to only one DC To fully facilitate Spark-C* connector data locality awareness, Spark workers should be collocated with Cassandra nodes 10
- 11. Spark Applications Deployment Revisited Cluster Manager: ● Spark Standalone ● YARN ● Mesos 11
- 12. Managing Cluster Resources: Mesos ● heterogenous workloads ● full cluster utilization ● static vs. dynamic resource allocation ● fault tolerance and disaster recovery ● single resource view at datacenter levelimage source: http://www.slideshare.net/caniszczyk/apache-mesos-at-twitter-texas-linuxfest-2014 12
- 13. Mesos Architecture Overview ● leader election and service discovery via ZooKeeper ● slaves publish available resources to master ● master sends resource offers to frameworks ● scheduler replies with tasks and resources needed per task ● master sends tasks to slaves 13
- 14. Bringing Spark, Mesos and Cassandra Together Deployment example ● Mesos Masters and ZooKeepers collocated ● Mesos Slaves and Cassandra nodes collocated to enforce better data locality for Spark ● Spark binaries deployed to all worker nodes and spark-env is configured ● Spark Executor JAR uploaded to S3 Invocation example spark-submit --class io.datastrophic.SparkJob /etc/jobs/spark-jobs.jar 14
- 15. Marathon ● long running tasks execution ● HA mode with ZooKeeper ● Docker executor ● REST API 15
- 16. Chronos ● distributed cron ● HA mode with ZooKeeper ● supports graphs of jobs ● sensitive to network failures 16
- 17. More Mesos frameworks ● Hadoop ● Cassandra ● Kafka ● Myriad: YARN on Mesos ● Storm ● Samza 17
- 18. Data ingestion: endpoints to consume the data Endpoint requirements: ● high throughput ● resiliency ● easy scalability ● back pressure 18
- 19. Akka features class JsonParserActor extends Actor { def receive = { case s: String => Try(Json.parse(s).as[Event]) match { case Failure(ex) => log.error(ex) case Success(event) => sender ! event } } } class HttpActor extends Actor { def receive = { case req: HttpRequest => system.actorOf(Props[JsonParserActor]) ! req.body case e: Event => system.actorOf(Props[CassandraWriterActor]) ! e } } ● actor model implementation for JVM ● message-based and asynchronous ● no shared mutable state ● easy scalability from one process to cluster of machines ● actor hierarchies with parental supervision ● not only concurrency framework: ○ akka-http ○ akka-streams ○ akka-persistence 19
- 20. Writing to Cassandra with Akka class CassandraWriterActor extends Actor with ActorLogging { //for demo purposes, session initialized here val session = Cluster.builder() .addContactPoint("cassandra.host") .build() .connect() override def receive: Receive = { case event: Event => val statement = new SimpleStatement(event.createQuery) .setConsistencyLevel(ConsistencyLevel.QUORUM) Try(session.execute(statement)) match { case Failure(ex) => //error handling code case Success => sender ! WriteSuccessfull } } } 20
- 21. Cassandra meets Batch Processing ● writing raw data (events) to Cassandra with Akka is easy ● but computation time of aggregations/rollups will grow with amount of data ● Cassandra is still designed for fast serving but not batch processing, so pre-aggregation of incoming data is needed ● actors are not suitable for performing aggregation due to stateless design model ● micro-batches partially solve the problem ● reliable storage for raw data is still needed 21
- 22. Kafka: distributed commit log ● pre-aggregation of incoming data ● consumers read data in batches ● available as Kinesis on AWS 22
- 23. Publishing to Kafka with Akka Http val config = new ProducerConfig(KafkaConfig()) lazy val producer = new KafkaProducer[A, A](config) val topic = “raw_events” val routes: Route = { post{ decodeRequest{ entity(as[String]){ str => JsonParser.parse(str).validate[Event] match { case s: JsSuccess[String] => producer.send(new KeyedMessage(topic, str)) case e: JsError => BadRequest -> JsError.toFlatJson(e).toString() } } } } } object AkkaHttpMicroservice extends App with Service { Http().bindAndHandle(routes, config.getString("http.interface"), config.getInt("http. port")) } 23
- 24. Spark Streaming 24 ● variety of data sources ● at-least-once semantics ● exactly-once semantics available with Kafka Direct and idempotent storage
- 25. Spark Streaming: Kinesis example val ssc = new StreamingContext(conf, Seconds(10)) val kinesisStream = KinesisUtils.createStream(ssc,appName,streamName, endpointURL,regionName, InitialPositionInStream.LATEST, Duration(checkpointInterval), StorageLevel.MEMORY_ONLY) } //transforming given stream to Event and saving to C* kinesisStream.map(JsonUtils.byteArrayToEvent) .saveToCassandra(keyspace, table) ssc.start() ssc.awaitTermination() 25
- 26. Designing for Failure: Backups and Patching ● be prepared for failures and broken data ● design backup and patching strategies upfront ● idempotece should be enforced 26
- 27. Restoring backup from S3 val sc = new SparkContext(conf) sc.textFile(s"s3n://bucket/2015/*/*.gz") .map(s => Try(JsonUtils.stringToEvent(s))) .filter(_.isSuccess).map(_.get) .saveToCassandra(config.keyspace, config.table) 27
- 28. The big picture 28
- 29. So what SMACK is ● concise toolbox for wide variety of data processing scenarios ● battle-tested and widely used software with large communities ● easy scalability and replication of data while preserving low latencies ● unified cluster management for heterogeneous loads ● single platform for any kind of applications ● implementation platform for different architecture designs ● really short time-to-market (e.g. for MVP verification) 29
- 30. Questions @antonkirillov datastrophic.io 30
Public clipboards featuring this slide
No public clipboards found for this slide