Storing time series data with Apache Cassandra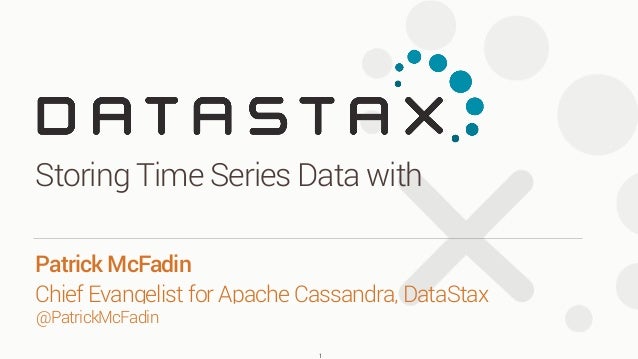


































































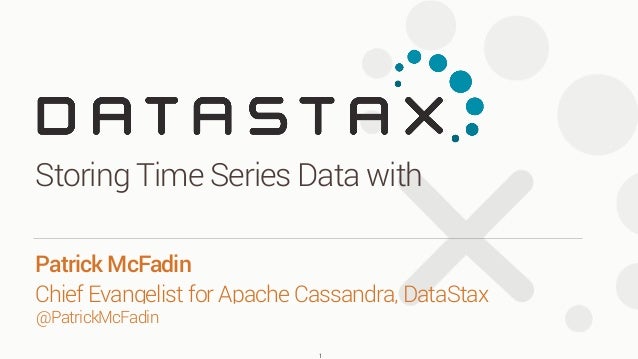
Upcoming SlideShare
Loading in …5
×
- 1. @PatrickMcFadin Patrick McFadin Chief Evangelist for Apache Cassandra, DataStax Storing Time Series Data with 1
- 2. My Background …ran into this problem
- 3. Gave it my best shot shard 1 shard 2 shard 3 shard 4 router client Patrick, All your wildest dreams will come true.
- 4. Just add complexity!
- 5. A new plan
- 6. Dynamo Paper(2007) • How do we build a data store that is: • Reliable • Performant • “Always On” • Nothing new and shiny Evolutionary. Real. Computer Science Also the basis for Riak and Voldemort
- 7. BigTable(2006) • Richer data model • 1 key. Lots of values • Fast sequential access • 38 Papers cited
- 8. Cassandra(2008) • Distributed features of Dynamo • Data Model and storage from BigTable • February 17, 2010 it graduated to a top-level Apache project
- 9. A Data Ocean or Pond., Lake An In-Memory Database A Key-Value Store A magical database unicorn that farts rainbows
- 10. Cassandra for Applications APACHE CASSANDRA
- 11. Basic Architecture
- 12. Row Column 1 Partition Key 1 Column 2 Column 3 Column 4
- 13. Partition Column 1 Partition Key 1 Column 2 Column 3 Column 4 Column 1 Partition Key 1 Column 2 Column 3 Column 4 Column 1 Partition Key 1 Column 2 Column 3 Column 4 Column 1 Partition Key 1 Column 2 Column 3 Column 4
- 14. Table Column 1 Partition Key 1 Column 2 Column 3 Column 4 Column 1 Partition Key 1 Column 2 Column 3 Column 4 Column 1 Partition Key 1 Column 2 Column 3 Column 4 Column 1 Partition Key 1 Column 2 Column 3 Column 4 Column 1 Partition Key 2 Column 2 Column 3 Column 4 Column 1 Column 2 Column 3 Column 4 Column 1 Column 2 Column 3 Column 4 Column 1 Column 2 Column 3 Column 4 Partition Key 2 Partition Key 2 Partition Key 2
- 15. Keyspace Column 1 Partition Key 1 Column 2 Column 3 Column 4 Column 1 Partition Key 2 Column 2 Column 3 Column 4 Column 1 Partition Key 1 Column 2 Column 3 Column 4 Column 1 Partition Key 1 Column 2 Column 3 Column 4 Column 1 Partition Key 1 Column 2 Column 3 Column 4 Column 1 Partition Key 2 Column 2 Column 3 Column 4 Column 1 Partition Key 2 Column 2 Column 3 Column 4 Column 1 Partition Key 2 Column 2 Column 3 Column 4 Column 1 Partition Key 1 Column 2 Column 3 Column 4 Column 1 Partition Key 2 Column 2 Column 3 Column 4 Column 1 Partition Key 1 Column 2 Column 3 Column 4 Column 1 Partition Key 1 Column 2 Column 3 Column 4 Column 1 Partition Key 1 Column 2 Column 3 Column 4 Column 1 Partition Key 2 Column 2 Column 3 Column 4 Column 1 Partition Key 2 Column 2 Column 3 Column 4 Column 1 Partition Key 2 Column 2 Column 3 Column 4 Table 1 Table 2 Keyspace 1
- 16. Node Server
- 17. Token Server •Each partition is a 128 bit value •Consistent hash between 2-63 and 264 •Each node owns a range of those values •The token is the beginning of that range to the next node’s token value •Virtual Nodes break these down further Data Token Range 0 …
- 18. Cluster Server Token Range 0 0-100 0-100
- 19. Cluster Server Token Range 0 0-50 51 51-100 Server 0-50 51-100
- 20. Cluster Server Token Range 0 0-25 26 26-50 51 51-75 76 76-100 Server ServerServer 0-25 76-100 26-5051-75
- 21. Replication 10.0.0.1 00-25 DC1 DC1: RF=1 Node Primary 10.0.0.1 00-25 10.0.0.2 26-50 10.0.0.3 51-75 10.0.0.4 76-100 10.0.0.1 00-25 10.0.0.4 76-100 10.0.0.2 26-50 10.0.0.3 51-75
- 22. Replication 10.0.0.1 00-25 10.0.0.4 76-100 10.0.0.2 26-50 10.0.0.3 51-75 DC1 DC1: RF=2 Node Primary Replica 10.0.0.1 00-25 76-100 10.0.0.2 26-50 00-25 10.0.0.3 51-75 26-50 10.0.0.4 76-100 51-75 76-100 00-25 26-50 51-75
- 23. Replication DC1 DC1: RF=3 Node Primary Replica Replica 10.0.0.1 00-25 76-100 51-75 10.0.0.2 26-50 00-25 76-100 10.0.0.3 51-75 26-50 00-25 10.0.0.4 76-100 51-75 26-50 10.0.0.1 00-25 10.0.0.4 76-100 10.0.0.2 26-50 10.0.0.3 51-75 76-100 51-75 00-25 76-100 26-50 00-25 51-75 26-50
- 24. Consistency DC1 DC1: RF=3 Node Primary Replica Replica 10.0.0.1 00-25 76-100 51-75 10.0.0.2 26-50 00-25 76-100 10.0.0.3 51-75 26-50 00-25 10.0.0.4 76-100 51-75 26-50 10.0.0.1 00-25 10.0.0.4 76-100 10.0.0.2 26-50 10.0.0.3 51-75 76-100 51-75 00-25 76-100 26-50 00-25 51-75 26-50 Client Write to partition 15
- 25. Consistency level Consistency Level Number of Nodes Acknowledged One One - Read repair triggered Local One One - Read repair in local DC Quorum 51% Local Quorum 51% in local DC
- 26. Consistency DC1 DC1: RF=3 Node Primary Replica Replica 10.0.0.1 00-25 76-100 51-75 10.0.0.2 26-50 00-25 76-100 10.0.0.3 51-75 26-50 00-25 10.0.0.4 76-100 51-75 26-50 10.0.0.1 00-25 10.0.0.4 76-100 10.0.0.2 26-50 10.0.0.3 51-75 76-100 51-75 00-25 76-100 26-50 00-25 51-75 26-50 Client Write to partition 15 CL= One
- 27. Consistency DC1 DC1: RF=3 Node Primary Replica Replica 10.0.0.1 00-25 76-100 51-75 10.0.0.2 26-50 00-25 76-100 10.0.0.3 51-75 26-50 00-25 10.0.0.4 76-100 51-75 26-50 10.0.0.1 00-25 10.0.0.4 76-100 10.0.0.2 26-50 10.0.0.3 51-75 76-100 51-75 00-25 76-100 26-50 00-25 51-75 26-50 Client Write to partition 15 CL= One
- 28. Consistency DC1 DC1: RF=3 Node Primary Replica Replica 10.0.0.1 00-25 76-100 51-75 10.0.0.2 26-50 00-25 76-100 10.0.0.3 51-75 26-50 00-25 10.0.0.4 76-100 51-75 26-50 10.0.0.1 00-25 10.0.0.4 76-100 10.0.0.2 26-50 10.0.0.3 51-75 76-100 51-75 00-25 76-100 26-50 00-25 51-75 26-50 Client Write to partition 15 CL= Quorum
- 29. Multi-datacenter DC1 DC1: RF=3 Node Primary Replica Replica 10.0.0.1 00-25 76-100 51-75 10.0.0.2 26-50 00-25 76-100 10.0.0.3 51-75 26-50 00-25 10.0.0.4 76-100 51-75 26-50 10.0.0.1 00-25 10.0.0.4 76-100 10.0.0.2 26-50 10.0.0.3 51-75 76-100 51-75 00-25 76-100 26-50 00-25 51-75 26-50 Client Write to partition 15 DC2 10.1.0.1 00-25 10.1.0.4 76-100 10.1.0.2 26-50 10.1.0.3 51-75 76-100 51-75 00-25 76-100 26-50 00-25 51-75 26-50 Node Primary Replica Replica 10.0.0.1 00-25 76-100 51-75 10.0.0.2 26-50 00-25 76-100 10.0.0.3 51-75 26-50 00-25 10.0.0.4 76-100 51-75 26-50 DC2: RF=3
- 30. Multi-datacenter DC1 DC1: RF=3 Node Primary Replica Replica 10.0.0.1 00-25 76-100 51-75 10.0.0.2 26-50 00-25 76-100 10.0.0.3 51-75 26-50 00-25 10.0.0.4 76-100 51-75 26-50 10.0.0.1 00-25 10.0.0.4 76-100 10.0.0.2 26-50 10.0.0.3 51-75 76-100 51-75 00-25 76-100 26-50 00-25 51-75 26-50 Client Write to partition 15 DC2 10.1.0.1 00-25 10.1.0.4 76-100 10.1.0.2 26-50 10.1.0.3 51-75 76-100 51-75 00-25 76-100 26-50 00-25 51-75 26-50 Node Primary Replica Replica 10.0.0.1 00-25 76-100 51-75 10.0.0.2 26-50 00-25 76-100 10.0.0.3 51-75 26-50 00-25 10.0.0.4 76-100 51-75 26-50 DC2: RF=3
- 31. Multi-datacenter DC1 DC1: RF=3 Node Primary Replica Replica 10.0.0.1 00-25 76-100 51-75 10.0.0.2 26-50 00-25 76-100 10.0.0.3 51-75 26-50 00-25 10.0.0.4 76-100 51-75 26-50 10.0.0.1 00-25 10.0.0.4 76-100 10.0.0.2 26-50 10.0.0.3 51-75 76-100 51-75 00-25 76-100 26-50 00-25 51-75 26-50 Client Write to partition 15 DC2 10.1.0.1 00-25 10.1.0.4 76-100 10.1.0.2 26-50 10.1.0.3 51-75 76-100 51-75 00-25 76-100 26-50 00-25 51-75 26-50 Node Primary Replica Replica 10.0.0.1 00-25 76-100 51-75 10.0.0.2 26-50 00-25 76-100 10.0.0.3 51-75 26-50 00-25 10.0.0.4 76-100 51-75 26-50 DC2: RF=3
- 32. Cassandra Query Language - CQL
- 33. Table CREATE TABLE weather_station ( id text, name text, country_code text, state_code text, call_sign text, lat double, long double, elevation double, PRIMARY KEY(id) ); Table Name Column Name Column CQL Type Primary Key Designation Partition Key
- 34. Table CREATE TABLE daily_aggregate_precip ( wsid text, year int, month int, day int, precipitation counter, PRIMARY KEY ((wsid), year, month, day) ) WITH CLUSTERING ORDER BY (year DESC, month DESC, day DESC); Partition Key Clustering Columns Order Override
- 35. Insert INSERT INTO weather_station (id, call_sign, country_code, elevation, lat, long, name, state_code) VALUES ('727930:24233', 'KSEA', 'US', 121.9, 47.467, -122.32, 'SEATTLE SEATTLE-TACOMA INTL A', ‘WA'); Table Name Fields Values Partition Key: Required
- 36. Select id | call_sign | country_code | elevation | lat | long | name | state_code --------------+-----------+--------------+-----------+--------+---------+-------------------------------+------------ 727930:24233 | KSEA | US | 121.9 | 47.467 | -122.32 | SEATTLE SEATTLE-TACOMA INTL A | WA SELECT id, call_sign, country_code, elevation, lat, long, name, state_code FROM weather_station WHERE id = '727930:24233'; Fields Table Name Primary Key: Partition Key Required
- 37. Update UPDATE weather_station SET name = 'SeaTac International Airport' WHERE id = '727930:24233'; id | call_sign | country_code | elevation | lat | long | name | state_code --------------+-----------+--------------+-----------+--------+---------+------------------------------+------------ 727930:24233 | KSEA | US | 121.9 | 47.467 | -122.32 | SeaTac International Airport | WA Table Name Fields to Update: Not in Primary Key Primary Key
- 38. Delete DELETE FROM weather_station WHERE id = '727930:24233'; Table Name Primary Key: Required
- 39. Collections Set CREATE TABLE weather_station ( id text, name text, country_code text, state_code text, call_sign text, lat double, long double, elevation double, equipment set<text> PRIMARY KEY(id) ); equipment set<text> CQL Type: For Ordering Column Name
- 40. Collections Set List CREATE TABLE weather_station ( id text, name text, country_code text, state_code text, call_sign text, lat double, long double, elevation double, equipment set<text>, service_dates list<timestamp>, PRIMARY KEY(id) ); equipment set<text> service_dates list<timestamp> CQL Type Column Name CQL Type: For Ordering Column Name
- 41. Collections Set List Map CREATE TABLE weather_station ( id text, name text, country_code text, state_code text, call_sign text, lat double, long double, elevation double, equipment set<text>, service_dates list<timestamp>, service_notes map<timestamp,text>, PRIMARY KEY(id) ); equipment set<text> service_dates list<timestamp> service_notes map<timestamp,text> CQL Type Column Name Column Name CQL Key Type CQL Value Type CQL Type: For Ordering Column Name
- 42. UDF and UDA User Defined Function CREATE OR REPLACE AGGREGATE group_and_count(text) SFUNC state_group_and_count STYPE map<text, int> INITCOND {}; CREATE FUNCTION state_group_and_count( state map<text, int>, type text ) CALLED ON NULL INPUT RETURNS map<text, int> LANGUAGE java AS ' Integer count = (Integer) state.get(type); if (count == null) count = 1; else count++; state.put(type, count); return state; ' ; User Defined Aggregate As of Cassandra 2.2
- 43. Example: Weather Station • Weather station collects data • Cassandra stores in sequence • Application reads in sequence
- 44. Queries supported CREATE TABLE raw_weather_data ( wsid text, year int, month int, day int, hour int, temperature double, dewpoint double, pressure double, wind_direction int, wind_speed double, sky_condition int, sky_condition_text text, one_hour_precip double, six_hour_precip double, PRIMARY KEY ((wsid), year, month, day, hour) ) WITH CLUSTERING ORDER BY (year DESC, month DESC, day DESC, hour DESC); Get weather data given •Weather Station ID •Weather Station ID and Time •Weather Station ID and Range of Time
- 45. Primary Key CREATE TABLE raw_weather_data ( wsid text, year int, month int, day int, hour int, temperature double, dewpoint double, pressure double, wind_direction int, wind_speed double, sky_condition int, sky_condition_text text, one_hour_precip double, six_hour_precip double, PRIMARY KEY ((wsid), year, month, day, hour) ) WITH CLUSTERING ORDER BY (year DESC, month DESC, day DESC, hour DESC);
- 46. Primary key relationship PRIMARY KEY ((wsid),year,month,day,hour)
- 47. Primary key relationship Partition Key PRIMARY KEY ((wsid),year,month,day,hour)
- 48. Primary key relationship PRIMARY KEY ((wsid),year,month,day,hour) Partition Key Clustering Columns
- 49. Primary key relationship Partition Key Clustering Columns 10010:99999 PRIMARY KEY ((wsid),year,month,day,hour)
- 50. 2005:12:1:10 -5.6 Primary key relationship Partition Key Clustering Columns 10010:99999 -5.3-4.9-5.1 2005:12:1:9 2005:12:1:8 2005:12:1:7 PRIMARY KEY ((wsid),year,month,day,hour)
- 51. Partition keys 10010:99999 Murmur3 Hash Token = 7224631062609997448 722266:13850 Murmur3 Hash Token = -6804302034103043898 INSERT INTO raw_weather_data(wsid,year,month,day,hour,temperature) VALUES (‘10010:99999’,2005,12,1,7,-5.6); INSERT INTO raw_weather_data(wsid,year,month,day,hour,temperature) VALUES (‘722266:13850’,2005,12,1,7,-5.6); Consistent hash. 128 bit number between 2-63 and 264
- 52. Partition keys 10010:99999 Murmur3 Hash Token = 15 722266:13850 Murmur3 Hash Token = 77 For this example, let’s make it a reasonable number INSERT INTO raw_weather_data(wsid,year,month,day,hour,temperature) VALUES (‘10010:99999’,2005,12,1,7,-5.6); INSERT INTO raw_weather_data(wsid,year,month,day,hour,temperature) VALUES (‘722266:13850’,2005,12,1,7,-5.6);
- 53. Data Locality DC1 DC1: RF=3 Node Primary Replica Replica 10.0.0.1 00-25 76-100 51-75 10.0.0.2 26-50 00-25 76-100 10.0.0.3 51-75 26-50 00-25 10.0.0.4 76-100 51-75 26-50 10.0.0.1 00-25 10.0.0.4 76-100 10.0.0.2 26-50 10.0.0.3 51-75 76-100 51-75 00-25 76-100 26-50 00-25 51-75 26-50 Client Read partition 15 DC2 10.1.0.1 00-25 10.1.0.4 76-100 10.1.0.2 26-50 10.1.0.3 51-75 76-100 51-75 00-25 76-100 26-50 00-25 51-75 26-50 Node Primary Replica Replica 10.0.0.1 00-25 76-100 51-75 10.0.0.2 26-50 00-25 76-100 10.0.0.3 51-75 26-50 00-25 10.0.0.4 76-100 51-75 26-50 DC2: RF=3 Client Read partition 15
- 54. Data Locality wsid=‘10010:99999’ ? 1000 Node Cluster You are here!
- 55. Writes CREATE TABLE raw_weather_data ( wsid text, year int, month int, day int, hour int, temperature double, dewpoint double, pressure double, wind_direction int, wind_speed double, sky_condition int, sky_condition_text text, one_hour_precip double, six_hour_precip double, PRIMARY KEY ((wsid), year, month, day, hour) ) WITH CLUSTERING ORDER BY (year DESC, month DESC, day DESC, hour DESC);
- 56. Writes CREATE TABLE raw_weather_data ( wsid text, year int, month int, day int, hour int, temperature double, PRIMARY KEY ((wsid), year, month, day, hour) ) WITH CLUSTERING ORDER BY (year DESC, month DESC, day DESC, hour DESC); INSERT INTO raw_weather_data(wsid,year,month,day,hour,temperature) VALUES (‘10010:99999’,2005,12,1,10,-5.6); INSERT INTO raw_weather_data(wsid,year,month,day,hour,temperature) VALUES (‘10010:99999’,2005,12,1,9,-5.1); INSERT INTO raw_weather_data(wsid,year,month,day,hour,temperature) VALUES (‘10010:99999’,2005,12,1,8,-4.9); INSERT INTO raw_weather_data(wsid,year,month,day,hour,temperature) VALUES (‘10010:99999’,2005,12,1,7,-5.3);
- 57. Write Path Client INSERT INTO raw_weather_data(wsid,year,month,day,hour,temperature) VALUES (‘10010:99999’,2005,12,1,7,-5.3); Column 1 Partition Key 1 Column 2 Column 3 Column 4 Column 1 Partition Key 1 Column 2 Column 3 Column 4 Memtable SSTable SSTable SSTable SSTable Node Commit Log Data * Compaction *
- 58. Date Tiered Compaction Strategy •Group similar time blocks •Never compact again •Used for high density SSTable SSTable SSTable T=2015-01-01 -> 2015-01-5 T=2015-01-06 -> 2015-01-10 T=2015-01-11 -> 2015-01-15
- 59. Storage Model - Logical View 2005:12:1:10 -5.6 2005:12:1:9 -5.1 2005:12:1:8 -4.9 10010:99999 10010:99999 10010:99999 wsid hour temperature 2005:12:1:7 -5.3 10010:99999 SELECT wsid, hour, temperature FROM raw_weather_data WHERE wsid=‘10010:99999’ AND year = 2005 AND month = 12 AND day = 1;
- 60. 2005:12:1:10 -5.6 -5.3-4.9-5.1 Storage Model - Disk Layout 2005:12:1:9 2005:12:1:8 10010:99999 2005:12:1:7 Merged, Sorted and Stored Sequentially SELECT wsid, hour, temperature FROM raw_weather_data WHERE wsid=‘10010:99999’ AND year = 2005 AND month = 12 AND day = 1;
- 61. 2005:12:1:10 -5.6 2005:12:1:11 -4.9 -5.3-4.9-5.1 Storage Model - Disk Layout 2005:12:1:9 2005:12:1:8 10010:99999 2005:12:1:7 Merged, Sorted and Stored Sequentially SELECT wsid, hour, temperature FROM raw_weather_data WHERE wsid=‘10010:99999’ AND year = 2005 AND month = 12 AND day = 1;
- 62. 2005:12:1:10 -5.6 2005:12:1:11 -4.9 -5.3-4.9-5.1 Storage Model - Disk Layout 2005:12:1:9 2005:12:1:8 10010:99999 2005:12:1:7 Merged, Sorted and Stored Sequentially SELECT wsid, hour, temperature FROM raw_weather_data WHERE wsid=‘10010:99999’ AND year = 2005 AND month = 12 AND day = 1; 2005:12:1:12 -5.4
- 63. Read Path Client Column 1 Partition Key 1 Column 2 Column 3 Column 4 Column 1 Partition Key 1 Column 2 Column 3 Column 4 Memtable SSTable SSTable SSTable Node Data SELECT wsid,hour,temperature FROM raw_weather_data WHERE wsid='10010:99999' AND year = 2005 AND month = 12 AND day = 1 AND hour >= 7 AND hour <= 10;
- 64. Query patterns • Range queries • “Slice” operation on disk Single seek on disk 10010:99999 Partition key for locality SELECT wsid,hour,temperature FROM raw_weather_data WHERE wsid='10010:99999' AND year = 2005 AND month = 12 AND day = 1 AND hour >= 7 AND hour <= 10; 2005:12:1:10 -5.6 -5.3-4.9-5.1 2005:12:1:9 2005:12:1:8 2005:12:1:7
- 65. Query patterns • Range queries • “Slice” operation on disk Programmers like this Sorted by event_time 2005:12:1:10 -5.6 2005:12:1:9 -5.1 2005:12:1:8 -4.9 10010:99999 10010:99999 10010:99999 weather_station hour temperature 2005:12:1:7 -5.3 10010:99999 SELECT weatherstation,hour,temperature FROM temperature WHERE weatherstation_id=‘10010:99999' AND year = 2005 AND month = 12 AND day = 1 AND hour >= 7 AND hour <= 10;
- 66. Thank you! Bring the questions Follow me on twitter @PatrickMcFadin
Public clipboards featuring this slide
No public clipboards found for this slide